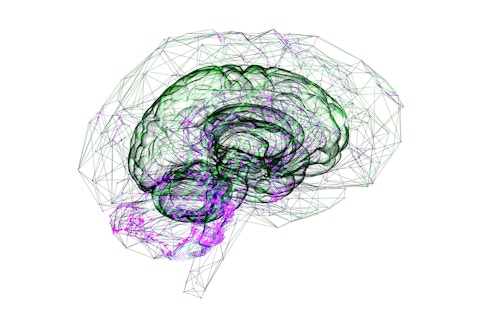
Individuals diagnosed with autism spectrum disorder (ASD) have a few core characteristics in common. But the nature and severity of these traits vary substantially from one individual to another, hence the term “spectrum.” This variation in ASD is found not only at the level of behavior but also in ASD genetics and brain anatomy (An and Claudianos, Neurosci. Biobehav. Rev., 2016; Hong et al., Biol. Psychiatry, 2020; Zheng et al., Autism Res., 2020) — making it challenging for researchers to pinpoint relationships between ASD-specific changes in brain and behavior. Understanding neuroanatomical variability within ASD could help to explain individual differences in ASD traits, make diagnoses more specific and develop tailored interventions. In a recent study supported in part by a SFARI Pilot Award, SFARI Investigators Stefano Anzellotti and Joshua K. Hartshorne, along with their colleague Aidas Aglinskas, explored neuroanatomical variability in ASD and its relationship with ASD traits, using a data-driven approach (contrastive machine learning) to analyze brain imaging scans from a large number of individuals with ASD (Aglinskas et al., Science, 2022).
In this study, the researchers hypothesized that neuroanatomical variation specific to ASD could be obscured by other factors unrelated to ASD. For example, genetic and environmental factors cause the brain of one individual to differ from another, in the population as a whole, regardless of whether they have ASD (Gu and Kanai, Front. Hum. Neurosci., 2014). And differences in measurement techniques between types of brain scanners can also lead to variations being detected in studies of neuroanatomy (Auzias et al., IEEE J. Biomed. Health Inform., 2016). To hone in on neuroanatomical variability that is present only in those with ASD, the researchers applied contrastive machine learning to a large magnetic resonance imaging (MRI) dataset from the Autism Brain Imaging Data Exchange (ABIDE), which contains participants with ASD and those who are typically developing. This machine-learning approach was able to find patterns of variation in neuroanatomy that are specific to ASD, separate from variation shared by all participants. These ASD-specific patterns of variation were associated with ASD clinical features, as measured by scores on commonly used questionnaires such as the Vineland Adaptive Behavior Scales and the Autism Diagnostic Observation Schedule. On the other hand, variation common to the general population was more associated with nonclinical characteristics, such as age and gender.
The researchers next showed that the machine-learning model used with the ABIDE dataset could be applied in a different set of individuals with ASD — that is, the model generalized to a new and independent dataset (a critical test of any model). To test generalization, they applied the same machine-learning model to MRI data from a new group of participants from Simons Searchlight (available through SFARI Base). Similar to what was found with the ABIDE dataset, patterns of neuroanatomy specific to ASD correlated better with ASD characteristics, whereas patterns that were shared with typically developing participants correlated more with nonclinical characteristics such as age, gender and type of brain scanner. Since the SFARI dataset contained genetic information relevant to ASD, the researchers were also able to show that ASD-specific neuroanatomical variation correlated with certain copy number variations, namely, 16p11.2 deletion and duplication. Generalization from the ABIDE dataset to the Simons Searchlight dataset makes this machine-learning model potentially applicable in clinical settings, where a model previously trained on one set of individuals with ASD could be used to analyze information from new individuals.
Interestingly, the study found that variation in neuroanatomy within ASD did not cluster into distinct categories or subtypes; instead, variation was organized along continuous dimensions. This work begins to answer a longstanding question about whether individual differences within ASD are best understood as multiple discrete subtypes/categories (as in the Diagnostic and Statistical Manual of Mental Disorders) or as continuous dimensions (as in the National Institute of Mental Health’s Research Domain Criteria). Although the study found that continuous dimensions best capture variation in ASD-related neuroanatomy, the researchers pointed out that it does not rule out the possibility that discrete diagnostic categories best capture individual differences in other ASD-related measures, for example, genetic or functional brain connectivity data.
Overall, the results revealed ASD-specific variation in brain anatomy that, importantly, relates to individual differences in ASD characteristics and genetics. This work advances our understanding of individual differences within ASD and the ultimate goal of developing personalized ASD treatment approaches.
Reference(s)
Contrastive machine learning reveals the structure of neuroanatomical variation within autism.
Aglinskas A., Hartshorne J., Anzellotti S.