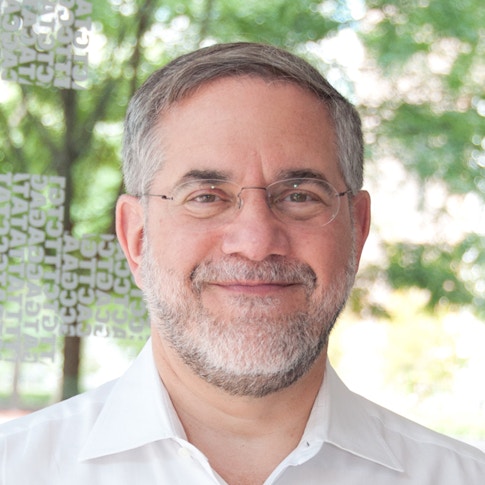
Animal models have long proved to be a valuable tool for studying the biological mechanisms of disease and uncovering potential treatments. To facilitate research into the links between genetics, neural and circuit mechanisms and behaviors in autism spectrum disorder (ASD), SFARI has established collaborations to create and distribute animal models of ASD risk alleles, including mouse, rat and zebrafish. Complementing these animal models, SFARI is creating human induced pluripotent stem cell (iPSC) lines from the Simons Simplex Collection and Simons Searchlight and is promoting research using postmortem brain tissue through Autism BrainNet. Each of these experimental systems holds value for increasing our understanding of mechanisms associated with ASD genetic variants, providing insights that can help shape clinical diagnoses and therapeutic developments. Each model, however, comes with its own strengths and limitations; understanding both is key to guiding decisions on what questions can and should be addressed with each system.
In a recent review article,1 Steven Hyman, director of the Stanley Center for Psychiatric Research at the Broad Institute of the Massachusetts Institute of Technology and Harvard University, discussed the benefits and limitations of mouse models for use in studying copy number variants (CNV) associated with neuropsychiatric conditions such as schizophrenia and ASD. In this article, Hyman expressed concerns with the field’s focus on “face validity” — how well a particular model system reflects chosen behavioral and biological features of a human disease — and “construct validity” — how biologically relevant the genetic changes are to the human disease being assessed.
I recently talked with Hyman about the utility and limitations of various ASD model systems and what role SFARI can play in helping to navigate the complexities of translating findings in model systems to an understanding of the mechanisms that underlie human neuropsychiatric conditions.
The interview has been edited for clarity and brevity.
You recently wrote a review article on the use of mouse models of CNV mutations to study neuropsychiatric conditions such as schizophrenia and ASD. Today, numerous model systems are available to researchers for studying neuropsychiatric disorders. In your view, what opportunities and potential issues do these animal models present for researchers?
Depending on the scientific question at hand, we now have multiple options. For example, neural cell types or brain organoids derived from human iPSCs permit experiments that require human genetic backgrounds. Diverse genome-engineered animal species, recently including nonhuman primates, allow experimental perturbations in the context of functioning brains. Much basic and translational neuroscience has relied on mouse and rat models and will continue to do so not only for reasons of cost and convenience, but also because there are powerful tools for altering both genomes and brains and a deep base of knowledge. That said, while various cellular and animal models can usefully model many molecular, cellular or behavioral mechanisms for translational neuroscience, we cannot expect to produce veridical models of human brain disorders in animals, and scientists must take care to avoid anthropomorphizing.
The development of treatments for depression is a good example of the types of pitfalls that can arise. Pharmacologic treatments for depression were discovered by serendipitous observation of antidepressant effects in humans in the absence of mechanistic insight. Attempts to discover additional antidepressants revolved around screening for compounds that would mimic the effects of the prototype antidepressant imipramine in rats, and later in mice, under certain laboratory conditions. One such screening approach involved a forced swim test, a test that involves placing a rat or mouse in water contained within a clear, high-walled cylinder from which the animal cannot escape. The rodent initially swims for a time and then becomes immobile, floating on the surface until removed. Imipramine reduces the rodent immobility time, but despite wide use, the forced swim test and similar assays have not led to the discovery of antidepressants with mechanisms that are fundamentally different from imipramine-like prototypes.
Why do I give this example? Because what was initially a black box drug screening assay came to be described in many papers as a test for depression-like behavior or even a model of depression and behavioral despair. This also led to the concepts of face and construct validity in disease models. Floating is most certainly not a veridical model of depression, whether we look from the point of view of mechanism, time course of onset, or symptoms. And this points to the need for more constrained descriptions of the strengths and weaknesses of our models or experimental systems with respect to their defined uses.
“Very diverse forms of causation can lead to the same cognitive or behavioral output. It’s important, and I really care that we get this right because we need to know the actual causes of these disorders if we are to develop disease-modifying interventions.”
You mentioned the terms “face validity” and “construct validity” just now and discuss these a fair bit in your article. You’ve argued that translational neuroscience would benefit from moving away from thinking along these lines. Could you clarify what “face validity” and “construct validity” mean and elaborate on your concerns with their use?
Face validity refers to the idea that you can plausibly relate the phenotype, such as behavior, that you are seeing in your model to features of the human condition being studied. Unfortunately, for human neurodevelopmental and neuropsychiatric disorders, the cognitive or behavioral features we might be looking at in these models are often many levels of complexity away from the underlying causes. Very diverse forms of causation can lead to the same cognitive or behavioral output. It’s important, and I really care that we get this right because we need to know the actual causes of these disorders if we are to develop disease-modifying interventions. The causal molecular mechanisms are what matter.
With construct validity, the idea is to make a model where one or more causal input factors are assumed to be the same as in the human disorder. It could be a genetic change. It could be an environmental exposure. On the surface, this sounds good. The trouble is that neuropsychiatric disorders have complex causes that are reflected by heterogeneous effects even at the levels of genes and environment. In neuropsychiatric disorders, even strongly acting disease-associated variants may not be fully penetrant on their own — meaning some individuals with these changes will not be affected — and may exhibit variable presentation where different individuals exhibit different features and challenges, occasionally warranting completely different clinical diagnoses. It is also increasingly clear that many other genes from the human background help determine penetrance and disease presentation. When we engineer even strongly acting disease-associated variants into a mouse, for example, the variant is now sitting within an entirely different genetic background from that of the affected human. So this sort of genome engineering can be highly informative with respect to gene function, developmental and cell type specific patterns of expression, and the like, but is that really construct validity? Have we produced a true model of the human disease in which we can freely interpret behavioral or cognitive alterations as relevant to the human disorder?
There is a problem with use of the word validity; what do face and construct validity accomplish conceptually? One of the hardest things we face in studying neuropsychiatric conditions is in knowing which questions to match with which models, and then integrating the information we gather across different models. By referring to model “validity,” we risk failing to question the model again. So our evaluation of what a model can teach us, and its meaning for understanding diseases and other conditions that warrant therapeutic attention becomes broken. Don’t get me wrong. We desperately need models, but we need to be asking more basic questions with these models. As a colleague of mine likes to say, we should look at these as models of mechanism not of a disease. And that’s very important because understanding mechanisms is what will power the systematic discovery of biomarkers and therapeutics.
As you’ve just mentioned, and as you raised in your review article, the genetic landscape of neuropsychiatric disorders is complex, reflecting the impact of numerous, partially penetrant, common genetic variants in addition to highly penetrant, rare variants. Yet the majority of neuropsychiatric disease animal models reflect disruptions to single, highly penetrant genes or genetic regions. How do we overcome this complexity when interpreting results from animal models?
One of the cardinal problems with neuropsychiatric disease models is that too often we study a single gene in a single inbred mouse strain. And precisely because genetic background does matter, the behaviors we see in a single strain may not be generalizable across mouse species let alone to humans. There’s a really lovely paper2 from Abraham Palmer’s lab where he examined two strong effect mutations on 30 different inbred mouse backgrounds — and the phenotypes they saw came and went in different strains, and even reversed their direction in some cases. So while it’s expensive and might not feel innovative, we need to repeat such experiments in two or three different strains to make sure that the phenotypes we see are likely to be generalizable across mice at least.
SFARI held a workshop to discuss the unique contributions that rats can bring to ASD research, and is supporting the creation and phenotyping of outbred transgenic rat models. What role do you see rat models playing in understanding genotype-phenotype relationships?
Genetic mouse models are still largely constructed in inbred strains, although outbred strains are increasingly being used. This use of genetically identical animals has the benefit of reducing the number of uncontrolled variables but also creates the generalizability problems we just discussed. Outbred rats tend to be healthier and more robust than inbred mouse strains, typically being free of the sensory and cognitive deficits often observed in inbred mice. Such animals also have heterogeneous genetic backgrounds. This increases the variability of the effects one might see, but means we are observing the effects of the transgene against diverse genetic backgrounds, which replicates the situation in humans.
“The important thing is to know what questions we should ask in each model and then compare and integrate data types across experimental systems in a rigorous manner.”
As you say, genetic background matters. You’ve also argued that the translational utility of animal models must be grounded in an understanding of how biological mechanisms relate between humans and these model systems. It seems like one way to address these points is through the use of systems that maintain aspects of the human genetic environment — using iPSC models and organoids, and post-mortem brain tissue, right?
Absolutely. To put genetic model data into context, we need to understand what a genetic variant of interest does within relevant human genetic backgrounds. Work in iPS cells, organoid systems and post-mortem brain tissue can give us these insights by comparing cell lines derived from people with the disorder to people without or comparing lines from individuals with very different clinical presentations. We can also use particular human cell lines as isogenic backgrounds against which to study engineered variants. Imaging work in humans, such as structural and functional magnetic resonance imaging (MRI), also allows direct analyses of human brains, but it’s very hard to relate genomic effects across many levels of complexity to the aggregate functioning of millions of neurons — which is what we see with MRI. In the end, we need to know whether the mechanisms we have selected to study in our animal model systems are actually relevant to the human conditions we are interested in. So we have to integrate across different model systems and experimental human biology, something that will likely require new technologies and computational methods. The important thing is to know what questions we should ask in each model and then compare and integrate data types across experimental systems in a rigorous manner.
You’ve suggested that animal models have real potential for helping us understand disease mechanisms at molecular, cellular and circuit levels. Many studies in animal models also look at effects on behavior but, as you’ve noted, there is a natural human tendency to anthropomorphize when interpreting animal behaviors. In the past several years though, a variety of labs have been working on high resolution, quantitative behavioral phenotyping strategies that incorporate computer vision and machine learning behavioral classifications, such as those developed by SFARI Investigators Bob Datta3, David Anderson (and collaborator Ann Kennedy 4,5) and Bence Ölveczky6,7. What role do you feel these types of behavioral tracking systems can play in understanding gene-behavior relationships in rodent models of neuropsychiatric conditions?
I think that unbiased approaches based on machine learning are very promising. The tendency for anthropomorphizing and for pattern-finding is irresistible to humans. The approaches that my colleagues Bob Datta and Bence Ölveczky at Harvard and David Anderson at CalTech have pioneered hold a lot of promise. I don’t want to oversell these since it is still early days, but I’m hopeful about approaches such as these that remove the distorted cognitive overlay that results from anthropomorphizing and that don’t invite terms like validity. Our historical reliance on face validity came about from a focus on behavior that was untethered to underlying molecular, cellular, synaptic and circuit mechanisms in most cases. Unbiased approaches will put us in a much better position to understand what human disease risk genes are actually doing mechanistically. It will still be hard to link all of these steps from genes to cognition and behavior together, but it’s a far better platform from which to begin.
“We cannot make progress without model systems. We just need to be very clear about what the utility of each model is and not inflate the lessons learned from them.”
You conclude your recent article by saying that mouse models present the field with challenges, but that there are steps that can be taken to strengthen their translational value. What role do you feel SFARI can play in supporting the translational value of the various disease models we’ve been discussing?
As I said at the start, I think that our current approach, based on relatively unconstrained assertions of validity, is broken. We need to work towards more nuanced and quantitative descriptions for the models that we are using. All of these models have utility and choosing to interpret these models rigorously will only enhance their value to the scientific community and ultimately to people in need of treatment. As a first step towards more rigorous interpretations, I think SFARI, together with partners you might invite, should host an initial meeting to map out better ways to think about, and report on, what each type of model can really tell us. I could see the value of SFARI spearheading these discussions, as SFARI is already putting a lot of effort and resources into developing mouse, rat, zebrafish and iPSC models of neurodevelopmental disorders. We cannot make progress without model systems. We just need to be very clear about what the utility of each model is and not inflate the lessons learned from them.
References
- Hyman S.E. Curr. Opin. Genet. Dev. 68, 99-105 (2021) PubMed
- Sittig L.J. et al. Neuron 91, 1253-1259 (2016) PubMed
- Wiltschko A.B. et al. Neuron 88, 1121-1135 (2015) PubMed
- Hong W. et al. Proc. Natl. Acad. Sci. USA 112, E5351-5360 (2015) PubMed
- Segalin W. et al. ELife 10, e63720 (2021) PubMed
- Marshall J.D. et al. Neuron 109, 420-437 (2021) PubMed
- Dunn T.W. et al. Nat. Methods 18, 564-573 (2021) PubMed