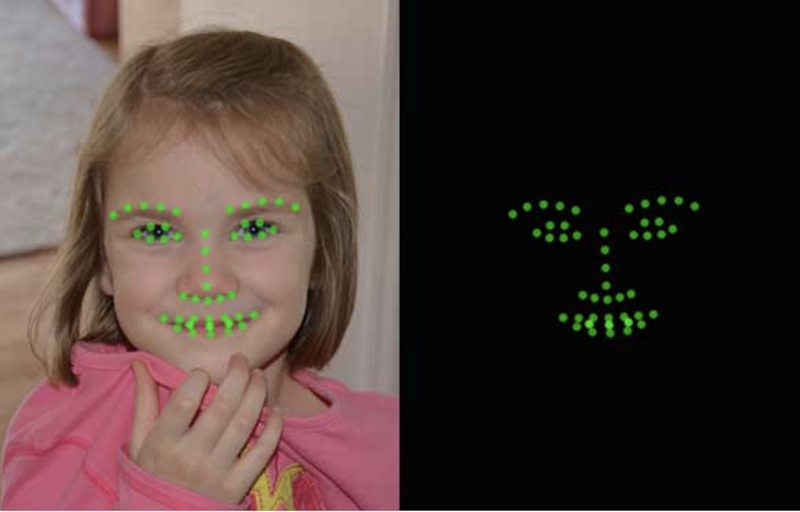
Scientists have made great strides in uncovering the genetic basis of autism spectrum disorder (ASD), but they still lack objective measures to assess its core symptoms — social and communication deficits and restricted and repetitive patterns of behaviors. The dearth of quantitative measures has significantly impacted ASD clinical research by making it difficult to evaluate baseline and treatment outcome in clinical trials and to stratify the autism population into biologically meaningful subgroups. The inability to group affected individuals into such subgroups is particularly important to develop targeted interventions in ASD, which encompasses a very broad genotypic and phenotypic spectrum.
To help accelerate the development of quantitative measures in autism research, SFARI launched a targeted request for applications (RFA) in 2015, which funded several projects that employ digital technologies to study autism behaviors. Three years later, on February 22, 2018, SFARI continued the conversation on digital phenotyping at a workshop that discussed using digital tools to reproducibly and accurately characterize behavior in neurodevelopmental and psychiatric disorders, including ASD.
As the development of those technologies requires rigorous testing and large-scale data, one goal of the workshop was to consider how the tens of thousands of individuals with ASD and their first-degree family members enrolled in SPARK (Simons Foundation Powering Autism Research for Knowledge)1 may provide a valuable resource for data collection. All these participants are re-contactable online, thus making the SPARK project well poised to help researchers in these endeavors.
Speakers at the workshop included Tom Insel (MindStrong Health), Nicholas Allen (University of Oregon), George Roussou (Birkbeck College, University of London), Guillermo Cecchi (IBM Research), Stephan Scherer (Embodied, Inc.), Laura Germine (McLean Hospital, Harvard Medical School) and SFARI Investigators Robert Schultz (University of Pennsylvania) and Guillermo Sapiro (Duke University).
In addition, Cori Bargmann (The Rockefeller University, Chan Zuckerberg Initiative), Jamie Hamilton (Michael J. Fox Foundation), Lara Mangravite (Sage Bionetworks), Stewart Mostofsky (Kennedy Krieger Institute), SFARI Investigator Elise Robinson (Harvard T. Chan School of Public Health, Broad Institute) and Catalin Voss (Stanford University) joined the conversation as external discussants.
“Digital phenotype is a new kind of biomarker.”
- Tom Insel
Digital phenotyping: A new biomarker?
The day opened with a presentation by Tom Insel, who discussed the use of digital technologies in clinical assessment. Insel argued that, traditionally, cognition, mood and behavior have been evaluated through approaches that are subjective, episodic, provider-based and reactive, but that in order to accelerate diagnosis and treatment, measures that are objective, continuous, ubiquitous and proactive are needed.
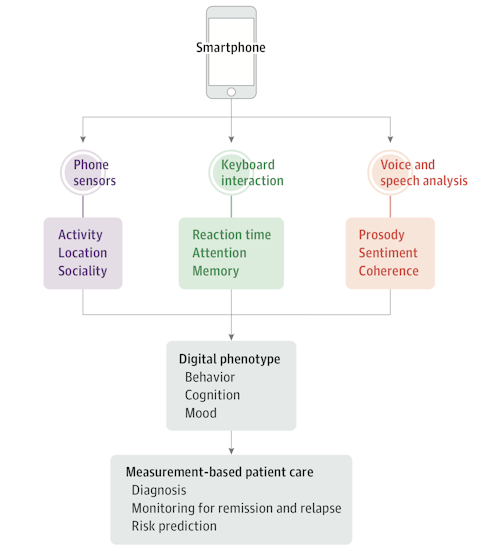
To Insel, such measures could be easily acquired by leveraging the technology that (almost) everybody already has in their pockets — smartphones. He argued that human-computer interactions such as keyboard strokes, taps and scrolls can be used to assess attention, processing speed and other executive functions; speech and voice recordings can reveal aspects of prosody, sentiment and coherence; and built-in sensors can track patterns of activity and location2 (Figure 1). Describing results from an initial study in which these digital measures were validated against gold-standard neuropsychological tests, he also showed that digital measurements may indeed help capture biologically meaningful instances of behavior3.
“Digital phenotype is a new kind of biomarker,” Insel says. “It is still in its early days, but the promise is real and actionable.”
Smartphone apps
Running passively in the background, smartphone-based apps can unobtrusively gather large amounts of information (and context) in the moment behavior occurs. This not only enhances the ecological validity of the data, but it also makes it possible to track symptom progression by assessing behavior continuously in between clinical visits. At the workshop, several apps for neuropsychological assessment were presented, the Effortless Assessment of Risk States (EARS) and the cloudUPDRS project.
The EARS app4, which was introduced by its developer, Nicholas Allen, collects data on an individual’s language, voice tone, facial expression, physiological activity, physical mobility and geolocation, with the aim to help to identify markers of affective state. Allen described its functionalities and highlighted potential applications to develop measures that can reliably indicate changes in psychological state and predict distress and suicide risk.
The cloudUPDRS is being developed by George Roussos, with the goal to provide unsupervised measurements of motor behaviors such as gait and tremor in Parkinson’s disease. Based on Part III of the Unified Parkinson’s Disease Rating Scale, the app helps to monitor symptoms and identify diagnostic tests that are most predictive of the user’s overall performance. Roussos argued that the information gathered by this app will aid researchers to develop finer-grained, individualized forms of analysis and interventions of Parkinson’s disease.
Computational approaches to neuropsychiatric disorders
Alongside smartphone-based technology, another area of research that was discussed at the workshop was the use of computational methods to quantify and monitor behavior and predict outcome in brain disorders.
Guillermo Cecchi showed that automated language programs and speech graphs can successfully discriminate between individuals with different types of psychosis (e.g., schizophrenia, mania) based on linguistic patterns extracted from the naturally uttered sentences of individuals with those disorders5 (Figure 2). Furthermore, he showed that high-level features such as ‘semantic coherence’ and ‘syntactic structure’ may predict the onset of psychosis in high-risk youths with accuracy greater than clinical ratings6.
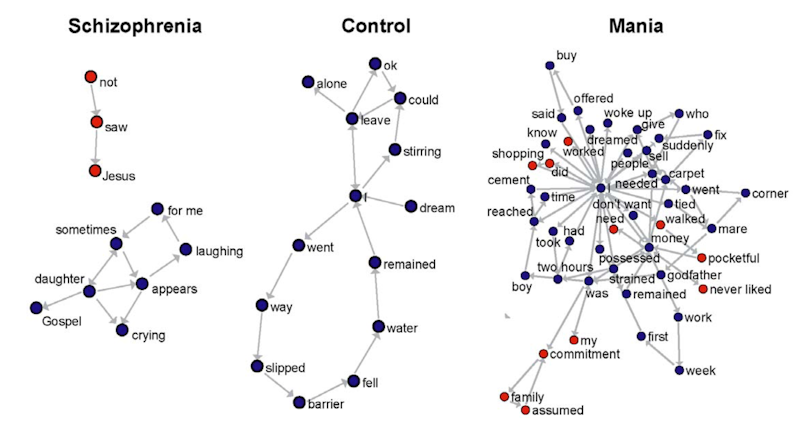
Also focusing on speech, Stefan Scherer then showed that an unsupervised machine learning algorithm can help identify individuals with and without depression from the analysis of simple acoustic features of the voice, like vowel space7 (Figure 3). These studies demonstrated that computational approaches may help advance clinical research by quantifying phenomena — like the fact people with depression tend to run the vowels when speaking — that had been previously described only qualitatively.
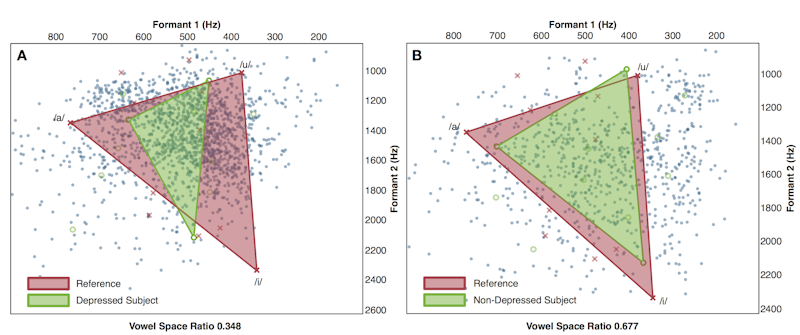
In addition to verbal markers, uses of quantitative approaches to characterize and measure nonverbal aspects of behavior, such as emotional and psychological states, were also discussed. In his presentation, Scherer described his research using MultiSense, a technology that can automatically detect distress associated with anxiety, depression and post-traumatic stress disorder from the analysis of the individual’s behaviors, including facial expressions, voice tone, and body fidgeting. Scherer showed that, by coupling MultiSense with a virtual human platform, SimSensei (Video 1), he could gather unbiased and multimodal information about individuals’ well-being that is consistent across participants — something the human eye, no matter how well trained, would never be able to do.
The importance of collecting multimodal information was also highlighted by Jeffrey Cohn, who discussed his research using automated analysis of face, gaze and head movement for clinical use. To this end, he developed 3-D face scans, which can be used to extract emotional affect (positive and negative) from measuring the amplitude and duration of movements of face muscles. The capacity to objectively analyze facial expressions may be especially important to study conditions such as depression and autism, where emotional affect may be compromised.
“Automatic assessments can augment what is being done. You could ask a therapist to count the number of smiles during a clinical assessment, but he or she will be able to do only that. Artificial agents, such as virtual humans or robots, could help gather unbiased and massive amounts of data,” Scherer says.
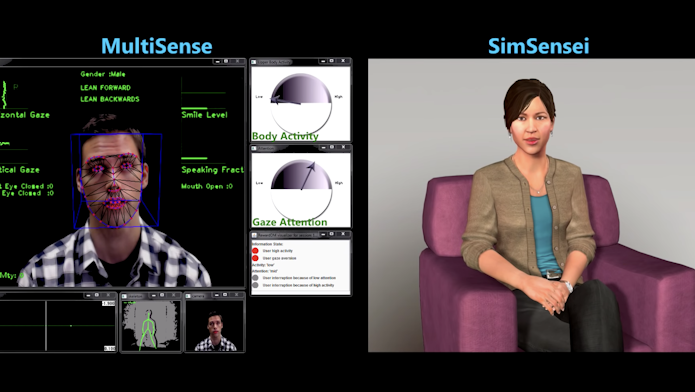
By clicking to watch this video, you agree to our privacy policy.
Web-based tools: Online, scalable phenotyping
The workshop also considered the advantages and challenges of using digital technologies to scale data collection by potentially making anyone with an internet connection a virtual research participant in the ‘digital lab.’
Illustrating this point, Laura Germine discussed TestMyBrain, a website she created to conduct cognitive testing online (e.g.,8,9). The website offers free tests for memory, perception and social skills and has provided data for more than 100 research studies and over 2 million participants since its launch in 2008. Germine argued that with nearly half of the population having access to the internet, researchers could conceivably measure half of the people in the world, a massive increase to the relatively small sample size of many behavioral studies. But there needs to be sufficient incentive for people to participate, so it is crucial that researchers design studies and tools that can easily engage people. To this end, she suggested minimizing barriers to accessing research opportunities, returning results to participants and respecting their time.
“Build studies that are open, mobile and participant centered,” says Germine. “Empower the patient and family communities to be an army of scientists that can make meaningful discoveries and innovations.”
Digital tools for autism research
As researchers are relying more and more on digital/online phenotyping, how can these technologies be used to quantify behaviors in ASD? A couple of presentations illustrated computer-vision methods to acquire, analyze and screen social and emotional behaviors in ASD.
SFARI Investigator Robert Schultz presented data from his studies on social-motor learning, where he digitized every aspect of the participants’ behavior (e.g., head, face, eye movements, posture, gestures, speech) while they were engaging in three-minute get-to-know-you interviews. One aim of this research is to quantitatively assess how individuals synchronize their movements in dyadic interactions, a capacity he expects to be deficient in people with ASD. This kind of fine-grained analyses are currently lacking in the autism human research field. Schultz believes that assessing all of these social behaviors objectively will help dissect the nuances of the autism phenotype, which today are only assessed in a binary fashion, by the presence or absence of core symptoms.
“We spend all this money on brain imaging and then use a scale that is a 0 or 1 to describe behavior,” says Schultz. “It is now possible to digitize every behavior that experts observe, without lapses in attention or memory.”
Apps for autism research are also starting to be developed. For instance, SFARI Investigator Guillermo Sapiro illustrated Autism & Beyond, an iPhone app that tracks children’s facial expressions while watching videos that elicit social-emotional responses (Video 2). The app is part of a large-scale collaboration between Duke University and Apple’s ResearchKit and is meant to provide a screening tool for autism. The program has now enrolled more than 1,000 families, and the developers are piloting its use outside the United States, with the aim of providing underserved communities around the world with access to a screening tool for autism that does not require administration or evaluation by trained clinicians.
“All you need is a phone,” says Sapiro, emphasizing not only the possibility to collect instant, ecologically valid responses, but also the opportunity to reach populations that would otherwise be left out of the research landscape.
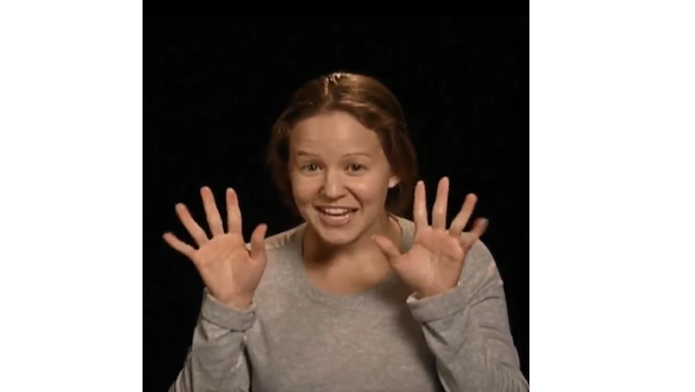
By clicking to watch this video, you agree to our privacy policy.
Future directions for SPARK
Digital tools show tremendous promise for clinical research. But there are challenges that researchers, and SPARK, would need to address before employing these methodologies. Data can be noisy, and analyses are computationally complex; machine learning algorithms still require better implementations. A major challenge in developing digital biomarkers is that large amounts of ‘ground-truth’ data are required, which typically involves labor-intensive manual annotation of data.
“...clinical researchers need better ways of quantifying language in individuals with ASD.”
- Pamela Feliciano
As there may not be a one-size-fits-all approach to ASD, it is important that digital tools can help identify clusters of phenotypes, so that researchers can develop clinical trials that stratify large segments of the population. And, most importantly, digital tools should ultimately be developed for actionable purposes. It isn’t enough to simply collect phenotypic data to label someone ‘at risk’; for digital tools to be useful in practice, they need to be part of a diagnostic tool or intervention, or applied in a new therapy.
“Think of SFARI as a sandbox,” says Insel. “We can use the 50,000 families they are recruiting to collect data and develop new ideas.”
With these lessons in mind, the first step for SPARK will be to identify those behaviors that can best serve as outcome measures in ASD research; next, the appropriate technologies to acquire those data can be designed and/or implemented. Since the workshop, SFARI has been considering several options, and it appears that the development of tools to automate language assessments may be one the most promising candidates.
“The discussions at the workshop made it clear that clinical researchers need better ways of quantifying language in individuals with ASD,” says Pamela Feliciano, SPARK Scientific Director and SFARI Senior Scientist. “Development of such tools is no small order, but the tens of thousands of participants in SPARK present a real opportunity to collect and analyze data at a scale that hasn’t been possible yet.”
References
- SPARK Consortium. Neuron 97, 488-493 (2018) PubMed
- Insel T.R. JAMA 318, 1215-1216 (2017) PubMed
- Dagum P. NPJ Digit. Med. 1 (2018) Article
- Lind M.N. et al. JMIR Ment. Health 5, e10334 (2018) PubMed
- Mota N.B. et al. PLoS One 7, e34928 (2012) PubMed
- Bedi G. et al. NPJ Schizophr. 1, 15030 (2015) PubMed
- Scherer S. et al. IEEE ICASSP (2015) Article
- Wilmer J.B. et al. Proc. Natl. Acad. Sci. USA 107, 5238-41 (2010) PubMed
- Germine L. et al. PLoS One 12, e0129612 (2015) PubMed