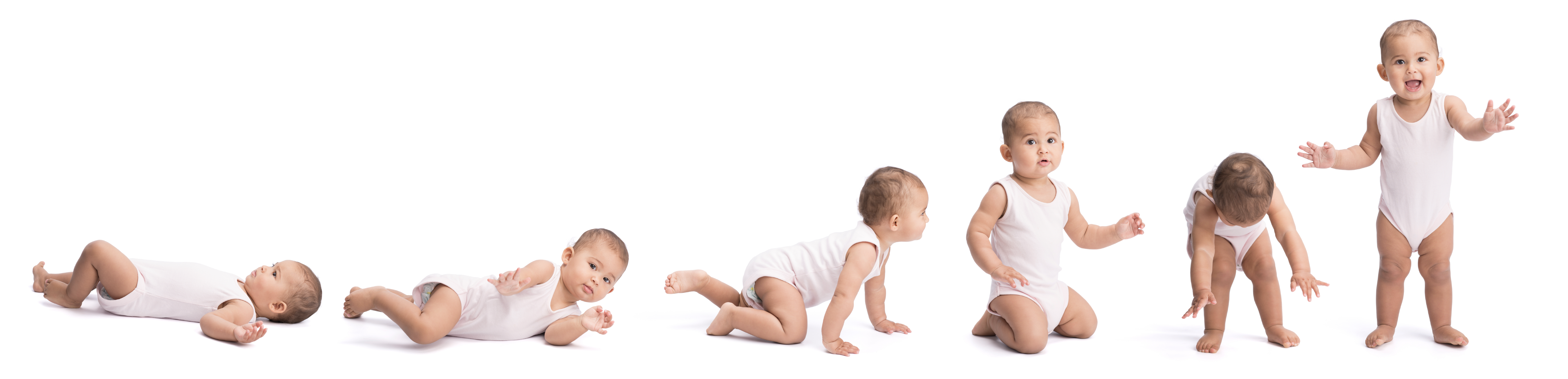
RuslanDashinsky / iStock
What are the earliest signs of autism? Do signs differ between genetically defined autism subtypes? What are cognitive milestones of early development for all children? Is there a way to study these aspects in animal models of autism?
These and other questions surfaced throughout a SFARI-sponsored workshop entitled “Next Steps in Infancy Research on Autism,” held on April 18, 2019. The workshop brought together 12 experts, including those who study child development but not autism, as well as clinicians, geneticists and epidemiologists. The ensuing conversations — part research updates, part brainstorms — touched on measurable aspects of infant development that might be sensitive enough, reliable enough and easy enough to use to help detect, parse and model autism.
“This is a meeting I’ve been dreaming about for so many years now,” said Elizabeth Spelke of Harvard University, who has been part of SFARI’s Scientific Advisory Board since 2011. Spelke studies the very beginnings of cognitive, social and emotional development in babies, and this research may inform ideas about how autism unfolds. She noted recent signs that make infancy research particularly opportune now: labs are joining together to get larger samples of infants; people are employing machine learning to aid interpretation of infant data; brain-imaging researchers have begun to conduct task-based experiments with infants; and web-based testing of infants has become a reality, which may ease data collection from these often wiggly creatures, as well as increasing participation from a larger number of subjects.
Another key development is SPARK (Simons Foundation Powering Autism Research for Knowledge), a project that is recruiting a cohort of 50,000 individuals with autism and their family members1. Wendy Chung, SPARK’s principal investigator and Director of Clinical Research at SFARI, said she was at the workshop to listen to the researchers’ priorities and needs. With genetic testing, she predicted that more homogeneous, genetically defined subgroups of autism will emerge. Elise Robinson, a genetic epidemiologist and co-organizer of the meeting, echoed this idea, saying that differences in early developmental trajectories may well map onto genetic subtypes of autism.
Moderated by Paul Wang, Deputy Director of Clinical Research at SFARI, the workshop included presentations and discussions from Elika Bergelson (Duke University), Somer Bishop (University of California, San Francisco), Alycia Halladay (Autism Science Foundation), Rebecca Landa (Kennedy Krieger Institute), Elise Robinson (Harvard T.H. Chan School of Public Health; Broad Institute of MIT and Harvard), Laura Schulz (Massachusetts Institute of Technology), Sarah Shultz (Marcus Autism Center; Emory University School of Medicine), Elizabeth Spelke (Harvard University), Shafali Spurling Jeste (University of California, Los Angeles), Audrey Thurm (National Institute of Mental Health), Henning Tiemeier (Harvard T.H. Chan School of Public Health) and Lonnie Zwaigenbaum (University of Alberta).
Early markers of risk for autism
A productive source for early signs of autism has been the Baby Siblings Research Consortium (BSRC). Begun 15 years ago and now including 25 sites, this project studies the baby siblings of children with autism, as they are at heightened risk for the disorder. From very early on, researchers monitor these children closely, and by 3 years of age, about 20 percent of them are diagnosed with autism2. Three talks from BSRC members gave research updates and an overview of their challenges in collecting and making sense of data from the very young.
Lonnie Zwaigenbaum highlighted BSRC findings that characterize a large proportion of those who eventually develop autism: a rapid increase in brain surface area at 6-12 months3 (Figure 1), functional connectivity differences at 6 months4 and parent-reported differences in sensory interests and motor abilities at 6 months5. BSRC studies have also clarified a regressive nature to autism in a majority of these children, marked by a loss or diminution of skills like directed gaze or vocalization. This suggests that a child’s deviation from a normal social-communication skill trajectory — something like a pediatrician’s growth chart — may eventually flag autism risk. Finally, he noted that autism diagnosis happens at different times within this group, and those diagnosed later have milder symptoms6.

Whether these baby sibling findings apply to the general population remains unclear. For example, would similar brain changes characterize nonfamilial cases? Would parents without direct experience of autism notice subtle signs found by parents who already have a child with autism? In discussion, Robinson noted that registry data from Nordic countries suggests a lower rate of recurrence in siblings, with only about 7 percent developing autism, compared to the BSRC’s 20 percent rate. While the reasons for this difference are unclear, it suggests that the BSRC findings may not necessarily apply to everyone.
“The motor system plays an important role in infant learning,” said Rebecca Landa, as she argued that basic motor skills set the stage for exploring the world and interacting with others. Combing through measures of posture, walking and grasping may deliver clues for motor delays in the run-up to autism. A recent BSRC report found that poor fine motor skills at 6 months distinguished at-risk siblings, whether or not they developed autism, and were associated with higher autism symptom severity at 3 years of age7; however, the measure fell short of specifically predicting autism. Motor delays can be overcome; for example, infants with delays in performing simple motor acts of grasping for an object at 6 months usually catch up by 10 months, but then display other types of grasping differences. This suggests that infants find alternative strategies, but also makes it important to study them early enough to detect signs of something amiss. Finally, Landa said that motor skills support vocalization; in her study group, one-third of the children had both a motor delay at 6 months and a communication delay at 18 months of age8.
In discussion, the issue of finding signs that can discriminate autism from other things arose. For example, while pulling an infant up to a sitting position, 90 percent of babies who later develop autism show a head lag at 6 months9 — but so do many children who don’t develop autism. Spelke also mentioned that motor skills may often rely on sensory and cognitive abilities, which may be domains to explore in order to clarify the roots of motor delays.
“This is a meeting I’ve been dreaming about for so many years now.”
- Elizabeth Spelke
How information courses through the brain’s networks is disrupted in autism, and this has spurred studies of brain activity in the very young. One way to do this is through noninvasive electroencephalography (EEG), which has been applied successfully in babies. Shafali Spurling Jeste gave a brief tour of EEG studies from the BSRC, which are difficult to compare because of their small sample sizes and differences in age, protocols, and analysis methods used. Still, Jeste identified some trends, noting differences between high-risk (has a sibling affected with autism) and low-risk (no affected sibling) children in the brain’s social- and language-processing networks. Resting state studies, which track spontaneous brain signals, can detect abnormal brain synchrony as young as 3 months of age10; however, what is found depends on how the data are processed, and Jeste stressed that the field needed to standardize its methods to get robust results. She also said that in addition to focusing on group differences in these signals, researchers should pay attention to individual outliers because they might indicate interesting subtypes of autism.
Reasoning that what captures the attention of a baby influences what it learns about, Sarah Shultz focused on quantitative methods to measure the moment-to-moment changes in looking. One method took advantage of the fact that typically developing toddlers largely look at the same regions while watching naturalistic scenes; how these regions vary over time can be depicted as an “attentional funnel” (Figure 2). Toddlers with autism often look outside of this funnel, and this difference can be quantified. To evaluate social aspects of looking, Shultz presented a way to measure a baby’s response to a live feed of his or her parent smiling. To assess not just looking but also engagement, Shultz monitors blinking; there is some evidence indicating that people inhibit blinking when they pay close attention to something, and this might help provide a better idea of what captures the attention of children with autism11.

Obtaining easily repeated measures may be key, as several people during the discussions stressed how a measure changes over time for an individual child may be more revealing than a snapshot of that measure at a certain time. While this would require more data than typically collected, some noted that parents themselves could provide indirect but reliable information on their child’s speech or social engagement because parents adapt their behavior according to the interest level or abilities of their child.
“Many of us who do research on children would never dream of doing research on children with autism because it’s hard enough to recruit children.”
– Laura Schulz
Inspirations from typical development
Spelke began a series of talks centered on typical child development, which provides a potentially useful framework for understanding how things go awry in developmental disorders like autism. With simple methods, such as measuring looking time, Spelke and her lab have charted the development of six different categories of knowledge in babies concerning objects, agents, persons, number, places and forms. “These are very early mechanisms that equip kids to learn about the world,” she said.
Remarkably, babies seem to tune in to abstract understandings of a situation; for example, 10-month-olds can distinguish harder goals from easier ones while watching scenes of animated shapes encountering different physical obstacles, like gaps, ramps or walls12. Spelke also said that comparable knowledge categories had been found in animals, ranging from nonhuman primates to fruit flies. This is attractive for thinking about animal models, but children with atypical development like autism or intellectual disability have not yet been tested with these paradigms. Spelke was also enthusiastic about finding ways to implement lab-based protocols into rich and noisy environments of a child’s home as a way to garner more data and to turn lab discoveries into useful interventions.
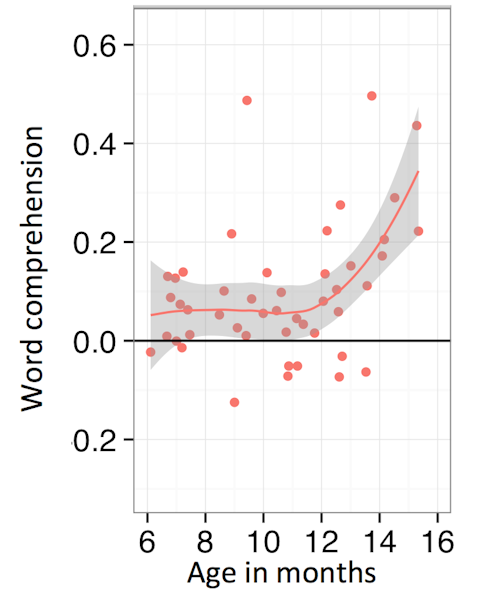
Autism is often first suspected when a child doesn’t begin to talk on time, but Elika Bergelson suggested that earlier phases of language development, such as learning to recognize sounds and attribute meaning to them, could provide earlier clues. Bergelson studies word comprehension in babies; when presented with two images, a baby will look at the image depicting the object whose name is spoken if the baby understands the word’s meaning. This has revealed that at 6 months, babies begin to understand common nouns; at 10 months, they begin to understand abstract words (like “uh-oh”); and at 14 months, their word comprehension surges13 (Figure 3). Through naturalistic audio and video data recorded from infants while at home, Bergelson showed that this surge is not driven by a sudden change in the types of things said to the baby at home14. Rather, babies seem to become better at taking advantage of their input, and this result illustrated an insight gained by combining at-lab data with in-home data.
It’s unclear whether autism is marked by difficulties in these early phases of language learning. In discussion, Zwaigenbaum noted that children with autism often have a reasonable understanding of words but struggle to use language appropriately; finding ways to measure pragmatic aspects of language usage may be more relevant. Sample sizes for language studies would need to grow considerably in order to understand the full range of variability and to capture those who have a language delay.
Laura Schulz began by emphasizing the heroic efforts it takes to recruit participants for autism studies. “Many of us who do research on children would never dream of doing research on children with autism because it’s hard enough to recruit children,” she said. To overcome this practical challenge, Schulz described an online platform for developmental research called Lookit, developed by Kim Scott in her lab15, 16 (Figure 4). The website hosts studies entirely online, which collect webcam video recordings from the participating families’ computers to gather data on children’s gazes, looking time or verbal responses. One study investigates children’s intuitive physics judgment, say for gravity; others have explored judgments of politeness or children’s sense of probability. Though recorded in often noisy home environments, Schulz said that the data are robust.
Lookit makes it easier for the family to return to the study as the child ages, giving precious longitudinal data. The ease of participation also means a larger number of participants, which gives power to measure small effects and, potentially, to increase the impact of an intervention. The Lookit participant pool is also much more representative of the U.S. population than a typical, lab-visiting sample, and the platform is now seeking to scale up to allow more researchers to recruit participants to their studies via this platform.

Something like Lookit could be a boon to autism researchers. Schulz said that participants might need to be brought into the lab once to obtain genetic or neurological data, but then afterward, they could complete studies online at home to give a richer characterization of their phenotypes. This scenario might be easier to fathom for time-strapped families who are often shuttling their special needs children from one appointment to the next.
Studying the general population in addition to autism cohorts
Henning Tiemeier argued there is still a place for longitudinal studies of the general population to gain insights on child development, including autism. Calling this approach ‘population neuroscience,’ he made the case with the Generation R cohort study, which follows 10,000 children and their mothers in Rotterdam, the Netherlands, from birth onward. The study retains about 80 percent of participants, making it well suited for picking up on early signals of disrupted development. Tiemeier highlighted some of Generation R’s autism-related findings: maternal thyroid hormone during pregnancy correlated with a child’s brain size and intelligence quotient 6 years later17; low motor tone in infancy was associated with autism symptoms 6 years later18; and an imaging study found that children with high scores on the Social Responsiveness Scale (SRS) had brain activity marked by longer times in a disconnected state at rest19. He acknowledged that it was difficult to keep children eventually diagnosed with autism in the study.
Taking a different tack, SFARI’s SPARK cohort is enrolling children with an autism diagnosis through 31 clinical sites in the U.S. or online. Chung made what she called an ‘unabashed advertisement’ of the cohort, which so far consists of more than 68,000 individuals with autism (including 17,700 trios, meaning a child affected by autism and both parents). Genetic data (whole-exome sequences and genome-wide genotyping data) for more than 5,000 trios is currently available to approved researchers via SFARI Base. Importantly, participants have agreed to be recontacted by researchers, which allows them to take part in new studies via SPARK’s research match program as well as continuing to provide longitudinal data via SPARK’s portal.
Looking forward
Jeste rounded out the presentations with a rundown of the BSRC’s next steps. Among these, one is to identify neural functions in early infancy that are precursors to atypical development, with an initial focus on EEG signals; a second is to study markers of resilience; a third is to see how markers of risk might predict response to an intervention. Jeste noted that BSRC children who develop autism often have better outcomes than those not in the study; if true, this ‘surveillance effect’ suggests that repeated contact with researchers may amount to an intervention. Jeste also said the group is moving to identify new risk groups, such as children with TSC1 mutations, 22q11.2 deletions or who spent their first days in neonatal intensive care units.
In discussion, people explored other potential risk groups that would be worth studying at young ages. Screens involving parent concerns, motor issues or eye-tracking anomalies might work, though people agreed that the degree of autism-specific enrichment in these groups was unclear.
Acknowledging the lack of causal links between the categories of knowledge identified by Spelke and behavioral outcomes, Wang asked the group whether these cognitive domains warranted study in animal models. Because something is known about the brain circuitry underlying numeracy and navigation, these two domains were suggested as initial test cases. Alongside this, however, Spelke and others urged that more human studies were necessary to track cognition in both normal development and autism.
SPARK was noted as a potential platform for ‘BSRC-lite’ types of studies because any new siblings born into a SPARK family could provide more prospective data on high-risk infants. The numbers and recontactability of the SPARK participant community may also help build the rich phenotypic data needed to characterize a child’s developmental trajectory and identify early markers of risk for autism.
References
- SPARK Consortium Neuron 97, 488-493 (2018) PubMed
- Ozonoff S. et al. Pediatrics 128, e488-e495 (2011) PubMed
- Hazlett H.C. et al. Nature 542, 348-351 (2017) PubMed
- Emerson R.W. et al. Sci. Transl. Med. 9, eaag2882 (2017) PubMed
- Sacrey L.R. et al. Autism 22, 322-334 (2018) PubMed
- Zwaigenbaum L. et al. Autism Res. 9, 790-800 (2016) PubMed
- Iverson J.M. et al. J. Abnorm. Psychol. 128, 69-80 (2019) PubMed
- Bhat A.N. et al. Infant Behav. Dev. 35, 838-846 (2012) PubMed
- Flanagan J.E. et al. Am. J. Occup. Ther. 66, 577-585 (2012) PubMed
- Levin A.R. et al. J. Neurodev. Disord. 9, 34 (2017) PubMed
- Shultz S. et al. Proc. Natl. Acad. Sci. USA 108, 21270-21275 (2011) PubMed
- Liu S. et al. Science 358, 1038-1041 (2017) PubMed
- Bergelson E. and Swingley D. Proc. Natl. Acad. Sci. USA 109, 3253-3258 (2012) PubMed
- Bergelson E. et al. Dev. Sci. 22, e12715 (2019) PubMed
- Scott K. and Schulz L. Open Mind 1, 4-14 (2017) Article
- Scott K. et al. Open Mind 1, 15-29 (2017) Article
- Korevaar T.I. et al. Lancet Diabetes Endocrinol. 4, 35-43 (2016) PubMed
- Serdarevic F. et al. Autism Res. 10, 757-768 (2017) PubMed
- Rashid B. et al. Hum. Brain Mapp. 39, 3127-3142 (2018) PubMed