Dysregulation of alternative splicing in autism spectrum disorder (ASD) has been implicated by studies of postmortem brain tissue and by the identification of de novo mutations that are predicted to affect splicing of particular mRNAs. The genetic evidence provided by the latter has been restricted to mutations that disrupt the canonical splice donor and acceptor sites that mark the ends of exons that are to be spliced together. Additional sequences, however, are known to influence splicing and could represent a larger set of targets of mutation that confer substantial risk of ASD.
To identify additional pathogenic splicing mutations (so-called ‘cryptic splice mutations’), Kyle Kai-How Farh and investigators at Illumina, in collaboration with SFARI Investigator Stephan Sanders and others, developed a deep-learning approach called SpliceAI. This neural network was trained on well-annotated genomic data from the GENCODE project and derived information from 10 kilobases surrounding each nucleotide to be tested for a role in splicing. They found that this method had high power to predict splice junctions on arbitrary pre-mRNA sequences, markedly outperforming previous approaches. Predicted splicing events in genomic data from the GTEx cohort were validated by RNA sequencing, and the authors used the large ExAC and gnomAD population control databases to show that these cryptic splice mutations are strongly deleterious.
The authors used SpliceAI to predict splice mutations in the Deciphering Developmental Disorders cohort, the Simons Simplex Collection (SSC) and the cohort of the Autism Sequencing Consortium. All told, they calculated that cryptic splice mutations contribute to 9-11 percent of cases of neurodevelopmental disorders, which constitutes a major new class of risk mutations in ASD. Finally, they carried out RNA sequencing of lymphoblastoid cell lines derived from individuals in the SSC to show that 21 of 28 (75 percent) predicted splice mutations actually disrupt splicing of the associated transcript. Given the power of this deep-learning approach, cryptic splice mutations should be screened for in all developing ASD cohorts, such as SPARK.
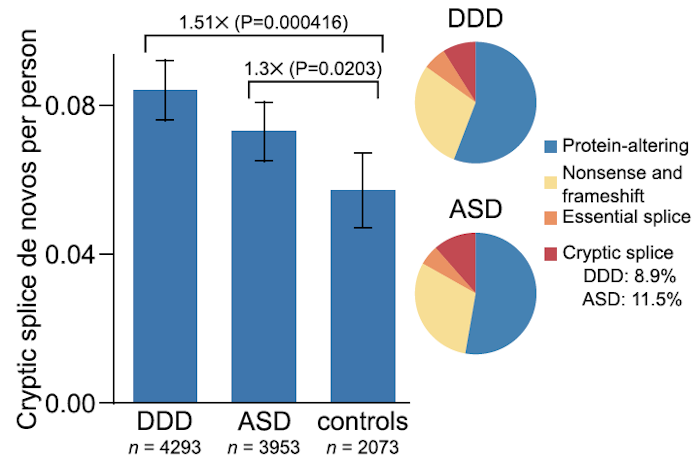
Reference(s)
Predicting splicing from primary sequence with deep learning.
Jaganathan K., Kyriazopoulou Panagiotopoulou S., McRae J.F., Darbandi S.F., Knowles D., Li Y.I., Kosmicki J.A., Arbelaez J., Cui W., Schwartz G.B., Chow E.D., Kanterakis E., Gao H., Kia A., Batzoglou S., Sanders S., Farh K.K.