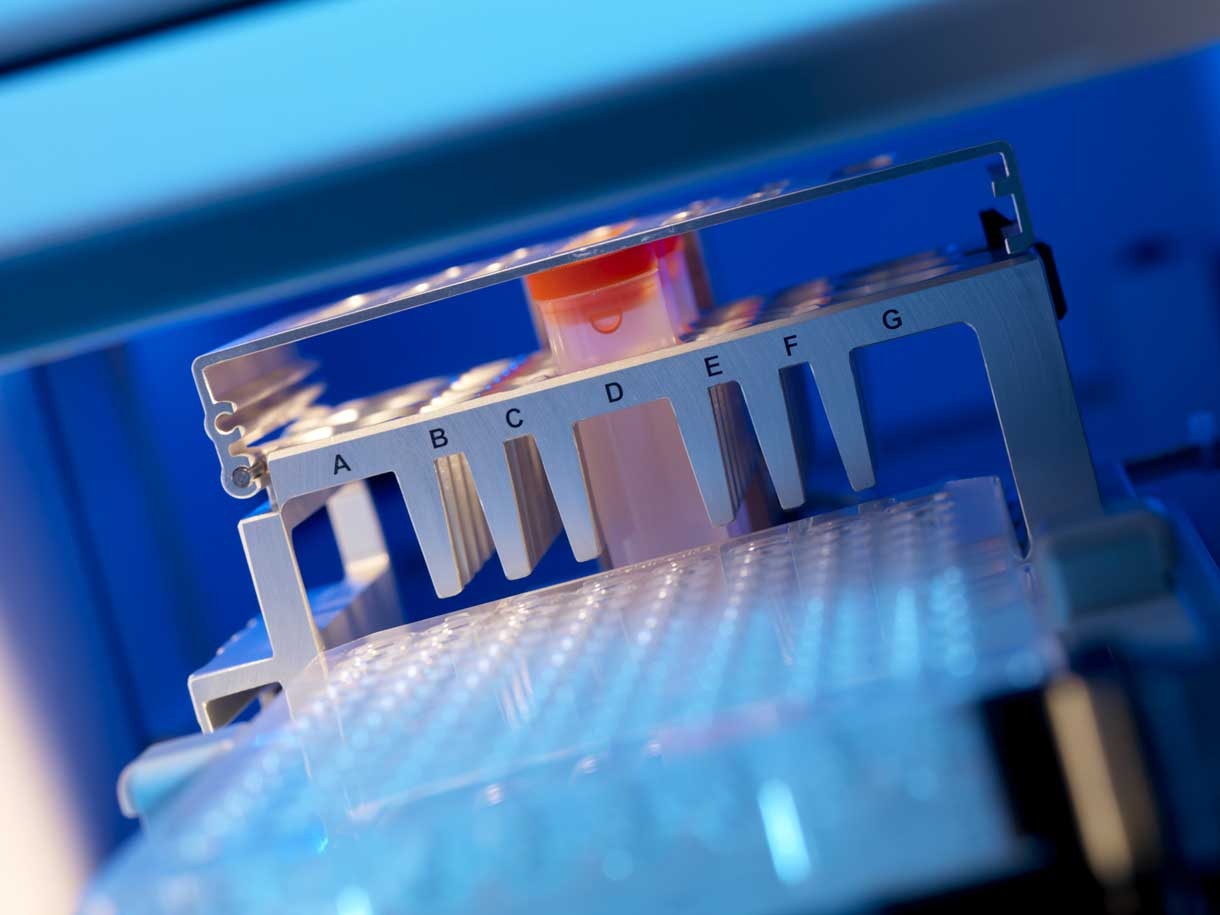
Andrew Brookes, National Physical Laboratory/Science Source
High-throughput biology: High-throughput screening approaches are being developed to test the functional effects of de novo missense variants identified in the Simons Simplex Collection (SSC) and other autism cohorts.
A recent landmark report in Neuron identified 71 loci in the genome that confer risk for autism spectrum disorder (ASD), underscoring the dramatic progress that geneticists have made in clarifying the genetic architecture of the disorder1. This was made possible by an integrated analysis of exome sequencing data from the Simons Simplex Collection (SSC) and samples independently ascertained and sequenced by the Autism Sequencing Consortium2.
Despite this new high watermark for ASD genetics, there is still much to be learned from the SSC and other collections. For example, a previous analysis found that individuals with ASD are more likely to carry a de novo missense mutation (i.e., a point mutation in which a single nucleotide change results in a codon that encodes a different amino acid) than either a de novo gene-disrupting mutation or a de novo copy number variant3. Given that only about 13 percent of identified de novo missense variants actually contribute to risk for ASD3, this creates a challenging situation and requires that additional work be done on this largest class of ASD-associated mutations to identify the subset that is pathogenic.
SFARI targeted awards for screening variants
In light of this challenge, the Simons Foundation Autism Research Initiative (SFARI) announced the Functional Screen of Autism-Associated Variants request for applications (RFA) in 2015. We sought proposals for the development and application of medium- and high-throughput screens to test the functional effects of de novo missense variants identified in the SSC and other collections. Five proposals were selected for funding (for more details, see the award announcement):
- George Church, Ph.D. (Harvard Medical School)
Human gene editing and in situ sequencing of neuronal microcircuit arrays - Kurt Haas, Ph.D. (University of British Columbia)
A multi-platform approach to the functional assessment of autism gene variants - Paul Sternberg, Ph.D. (California Institute of Technology)
Analysis of autism-associated alleles in C. elegans - Michael Wangler, M.D., Shinya Yamamoto D.V.M., Ph.D. (Baylor College of Medicine)
In vivo functional analysis of autism candidate genes - Haiyuan Yu, Ph.D. (Cornell University), Bernie Devlin, Ph.D. (University of Pittsburgh), Kathryn Roeder, Ph.D. (Carnegie Mellon University)
Interactome perturbation by large-scale mutagenesis to find autism risk variants
We expect that these efforts will complement each other nicely, as they employ a range of model organisms (including C. elegans, Drosophila, zebrafish, mice and human neurons derived from induced pluripotent stem cells) in addition to diverse approaches (such as yeast two-hybrid high-throughput assays4 and neuronal microcircuit arrays). Given that there will inevitably be some overlap in the variants to be tested by each group, it’s our hope that a combined analysis may identify those that confer risk for ASD with high confidence and tell us more about the biological consequences of such mutations. Until that time, it’s worth taking note of an impressive series of recent papers in this area that reveal how much can be learned by tackling these variants of uncertain significance.
Functional evidence for missense variants contributing to autism
Two recent studies report a relatively straightforward but important use of functional assays to lend support to the pathogenicity of autism-risk alleles identified by exome or candidate-gene sequencing. The first identified an increased burden of missense variants in CTNND2, encoding delta-catenin, in ASD cases. In support of the statistical association, constructs carrying five such missense variants from probands with ASD (but none of the controls) failed to completely rescue readouts of CTNND2-dependent Wnt signaling in zebrafish where levels of CTNND2 had been reduced by morpholinos5. When transfected into rat hippocampal neurons, constructs harboring two CTNND2 missense variants also failed to replicate the dendritic spine density observed in neurons transfected with the wild-type allele.
Yet another recent study showed that functional assays of missense variants can be used not only to support claims of pathogenicity, but also to provide new biological insight into the regulation and mechanism of action of the protein. Mark Zylka and his colleagues reported that a de novo missense mutation in UBE3A disrupts a phosphorylation site for protein kinase A, which leads to enhanced UBE3A activity in vitro and increased turnover of UBE3A substrates in cell lines derived from an individual carrying this mutation7. Furthermore, excessive dendritic spine development — which previous studies have suggested may be related to autism pathogenesis — was observed in the cortex of mice expressing this missense mutation (Figure 2). Of note is that thisparticular missense mutation in UBE3A was identified in an individual with autism from the SSC, underscoring the ongoing value of SFARI’s flagship cohort and associated collection of biospecimens.
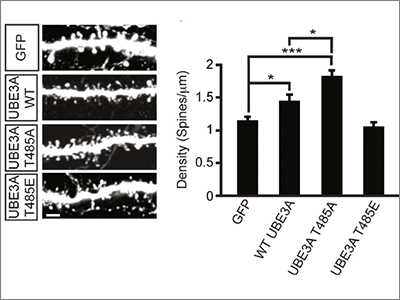
Missense variants beyond autism
In addition to the studies discussed above, two recent papers focusing on missense variants outside the context of ASD are worth mentioning, as they provide a broader view of the usefulness of this approach.
Heiko Runz and his colleagues were interested in rare missense alleles in LDLR, encoding the low-density lipoprotein receptor, which has an important influence on plasma LDL-cholesterol levels and risk of myocardial infarction8. The challenge they faced — a challenge faced by anyone doing rare-variant association studies — is a lack of statistical power given the large number of benign polymorphisms that are found in cases alongside the truly pathogenic variants. In their study, they report a scalable cell-based assay for cellular uptake of LDL, which enabled them to identify the functional LDLR alleles. By excluding the non-functional missense alleles from the statistical comparison of cases and controls, they were able to achieve a dramatic increase in power, revealing that a previously marginal association of LDLR missense variation with early-onset myocardial infarction is actually quite strong8.
Another notable study addressed the weaknesses of the currently available algorithms for predicting the pathogenicity of a missense variant — namely that such approaches do not take into account the genomic context of the allele. For example, a particular human missense variant might be predicted to be benign given the presence of the mutated allele in a number of other species. Some of these predictions will be incorrect, however, if the other species are protected by additional compensatory variants elsewhere in the gene. In an elegant analysis, Nicholas Katsanis and his colleagues focused on missense variants in two genes that were predicted to be benign by commonly used algorithms but are known to be functional through a variety of zebrafish assays of the sort already mentioned above9. Through these same assays, they then identified compensatory amino acid changes in other species that are not present in humans, and that rendered the mutated allele benign in those species9. If scaled up, this sort of analysis could reduce the number of ‘false-negative’ predictions made by the standard algorithms, and increase the yield of sequencing studies that focus on missense variants.
What about non-coding variants?
While much remains to be learned about the functional significance of missense mutations identified from exome sequencing, the increasing number of non-coding sequence variants identified as a result of genome-wide association studies (GWAS) and whole-genome sequencing analyses will necessitate the development of high-throughput functional screens that are also applicable for these variants. Matthew Freedman and his colleagues recently reported an experimental pipeline to enable the functional validation of such variants10. Their approach, known as CAUSEL (Characterization of Alleles USing Editing of Loci), involves an integrated pipeline in which candidate single-nucleotide polymorphisms (SNPs) are first evaluated by fine mapping, epigenomic profiling and epigenome editing, and then interrogated for causal function by using genome editing to create isogenic cell lines followed by phenotypic characterization. To demonstrate proof of concept, they established the functional significance of a non-coding variant in a previously identified prostate cancer risk locus located on chromosome 6q22.1. It will be interesting to see how widely applicable such a strategy is for establishing functional significance of other disease-associated SNPs.
In summary, it’s our hope that the functional screens sponsored under this SFARI RFA will lead to new platforms that can be used in the creative ways identified in these publications, and will enable the rapid characterization of the large ASD sequencing datasets — exome and genome — that are being sponsored by SFARI and other funding bodies.
References:
- Horev G. et al. Proc. Natl. Acad. Sci. USA 108, 17076-17081 (2011) PubMed
- Portmann T. et al. Cell Rep. 7, 1077-1092 (2014) PubMed
- Celen C. et al. Elife e25730 (2017) PubMed
- Bader P.L. et al. Proc. Natl. Acad. Sci. USA 108, 15432-15437 (2011) PubMed
- Poliak S. et al. J. Cell Biol. 162, 1149-1160 (2003) PubMed
- Peñagarikano O. et al. Cell 147, 235-246 (2011) PubMed
- McEvoy J.D. et al. Mol. Cell. Biol. 27, 3651-3656 (2007) PubMed
- Thompson B.J. et al. J. Exp. Med. 212, 953-970 (2015) PubMed
- Mervis C.B. et al. Am. J. Hum. Genet. 90, 1064-1070 (2012) PubMed
- Huang W.H. et al. Neuron 92, 392-406 (2016) PubMed
- Bozdagi O. et al. Mol. Autism 1, 15 (2010) PubMed
- Peça J. et al. Nature 472, 437-442 (2011) PubMed
- Clement J.P. et al. Cell 151, 709-723 (2012) PubMed
- Smith S.E. et al. Sci. Transl. Med. 3, 103ra197 (2011) PubMed
- Brunner D. et al. PLoS One 10, e0134572 (2015) PubMed
- Kabitzke P. et al. Genes Brain Behav. 17, 4-22 (2018) PubMed
- Wang X. et al. Hum. Mol. Genet. 20, 3093-3108 (2011) PubMed
- Wiltschko A.B. et al. Neuron 88, 1112-1135 (2015) PubMed