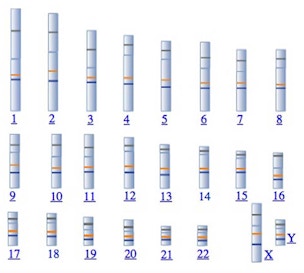
Imagine the following scenario: A physician sees a boy with delayed speech and diagnoses him with autism. Gold-standard clinical assessments — the Autism Diagnostic Observation Schedule and the Autism Diagnostic Interview-Revised — back up this diagnosis. An electroencephalography shows brain changes that suggest childhood epilepsy, although the boy has never had a seizure. Genetic testing of the chromosomes reveals no suspect duplications or deletions.
But, sequencing of SHANK3 — a gene that has been linked to autism — performed in a commercial laboratory, identifies a mutation in this gene: an insertion of a base pair in the eleventh coding exon, a region believed to code for protein. The mutation is predicted to lead to a frameshift — a shift of the DNA code that can create a premature stop when translated into protein.
The lab tells the boy’s parents that it has identified a “predicted disease-associated mutation.” Given the published evidence implicating SHANK3 in neurodevelopmental disorders, the prediction seems reasonable.
First, do no harm
The story is true, but the prediction almost certainly is not. The facts are laid out in a new paper in Brain Research from Joseph Buxbaum and colleagues and demonstrate that we need to use care when translating research findings into clinical practices.
Buxbaum and his colleagues found the same insertion in the boy’s unaffected mother when they sequenced SHANK3 in both parents. This is surprising, as SHANK3 mutations tend to be highly penetrant — they almost always lead to a phenotype.
Additional data in the paper shows why. It turns out that exon 11 probably does not code for protein. It is present in the standard human reference sequence RefSeq database. However, there are no matches to it in the expressed sequence tags database, which contains DNA copies of RNA messages for the genes that are expressed. It is also absent from the RefSeq databases for mice and rats.
The authors conclude that the human RefSeq database is in error. It is certainly not the only error, given the complexity of the human genome, but it highlights the danger in using research tools to inform decision-making in the doctor’s office.
This is not a new problem: similar difficulties exist in interpreting the results of genetic tests for other genes, such as BRCA1 for breast and ovarian cancer, and CFTR for cystic fibrosis.
The Brain Research paper includes a ‘position statement,’ which recommends that researchers establish a “large-scale collated database of genetic variation in individuals with and without neurodevelopmental disabilities,” and that they should provide guidelines that can be used by clinicians, genetic testing sites and families. This statement emerged from a conference held in Toronto in September on the translation of genetic discoveries into diagnostics.
Some databases on human genetic variation already exist, including dbSNP, dbVAR, the Human Gene Mutation Database, and the ever-growing 1000 Genomes Project. These databases are not yet adequate resources for clinical use, however. The international Human Variome Project may go a long way toward addressing many problems with such databases, as its mission is to make all genetic variation related to human health and disease accessible and as correct, transparent and comprehensive as possible.
Grappling with complexity:
At SFARI, we are faced with similar complexities when interpreting the genetic results generated by surveys of the Simons Simplex Collection — a genetic database of families that have only one child with autism. Most of the data generated are of uncertain clinical relevance, and we have set up committees of clinical geneticists to determine whether an individual’s personal physician should be notified of a particular finding. But, given the state of the science, these experts are simply making an educated judgment, not reaching a firm conclusion.
Although we are working to ensure that genetic databases at SFARI are error-free, difficulties in interpretation extend not only to the clinic, but to basic researchers as well. For example, the evolving SFARI Gene database, which was developed by Sharmila Basu and her colleagues at Mindspec, Inc., lists information for any gene implicated in autism in the literature. Basu and colleagues make no judgments about the strength of the evidence implicating a particular gene: they take all comers. This unbiased and comprehensive approach has a lot of merit as a first step, as it tells a user everything that has been reported.
But this can’t be the last step. There is always the danger that users who are new to the field of autism genetics will view the list as a set of genes with a confirmed role in autism susceptibility. Even for more knowledgeable users, the most useful resource would be a site that critically evaluates the evidence.
With this in mind, early in 2010 we recruited a group of early-career investigators to help us develop criteria to evaluate the strength of each candidate gene and present this information to the broader community. The first of these evaluations is expected to be available online in the first half of 2011.
In addition to Basu, our advisors include Brett Abrahams, Dan Arking, Dan Campbell, Heather Mefford, Eric Morrow and Lauren Weiss. Each of these young researchers has already made major contributions to the field.
We have learned many things, but the most telling — albeit not the most surprising — is that this process is exceedingly difficult. One obvious reason is that idiopathic autism is not a single-gene Mendelian disorder in which mutations segregate perfectly in families. As for all complex disorders, we need to consider the statistics and probability of risk for each allele.
A few of these statistical arguments lead to clear results, but for most the lines are fuzzy. For example, consider the case of a common gene variant. If it is overrepresented in people with autism compared with controls to a degree that is statistically significant — reaching a p-value of 10-8 — and this result is confirmed in another data set, then the conclusion is clear.
But what if a common variant is associated at a lesser degree of statistical strength, and is still independently replicated? Or, alternatively, what if the independent replication is in a different variant of the gene? What if it is not independently replicated, but other investigators have reported rare variants in that gene in individuals with autism? What if those rare variants look like they might be functional, but researchers have not sequenced enough control genomes to be sure that the variant actually affects the gene? What if there is evidence from postmortem brain tissue from an individual who had autism that this gene’s expression is altered?
How do you sensibly combine these lines of evidence? Does weak evidence in one area plus weak evidence in another area equal stronger evidence?
I could go on listing these permutations — and we have — but I trust the point is clear: there is no straightforward answer.
Guiding principles:
Given the elusive nature of the truth in evaluating genetically heterogeneous disorders such as autism, we have decided on a few basic guiding principles. First, we are putting the primary emphasis on evidence from human genetic studies, rather than functional ones. Many genomic variants may affect function, even to the point of causing seemingly relevant phenotypes in model organisms, but only a small number of these actually increase risk of the disorder in a human being.
Second, we plan to look at the evidence for each gene without prior assumptions about its strength. Sometimes the field believes that a particular gene is a risk factor, even though the evidence for this is surprisingly thin.
Finally, all of these criteria will be presented on the SFARI Gene website in a transparent manner — probably as a simple checklist. A summary of the relevant findings from the literature will appear alongside. We will then invite feedback from the community, and if the wisdom of the crowd identifies relevant literature that we have missed, or has a better interpretation of the data, the score for that gene will change. In this way we hope to arrive at a consensus that is based on the contributions of a larger group of interested researchers.
An additional outcome for this project is that it will suggest which experiments are needed to solidify the evidence for autism risk genes. Larger sample sizes, more comprehensive screening of controls and meta-analyses would all help boost confidence in the genes for which the evidence is currently quite weak — genes that are in the majority on the SFARI Gene list.
Our plan is to roll out the scores and annotations in 2011, and we invite your comments at that time.
It is important to note that this is not just an academic exercise. The case of the SHANK3 mutation mentioned above makes it clear that clinically relevant advice depends on the soundness of each link in the chain. Diagnosis and potential intervention will require not just that the identified mutation is valid, but that there is sufficient evidence that the gene harboring the mutation is actually involved in the disorder.
This is the the challenge ahead, not just for autism, but ultimately for all complex disorders in which genetic variation affects who is susceptible and who is not.